Understanding Semantic Segmentation Video: A Game Changer in Data Annotation
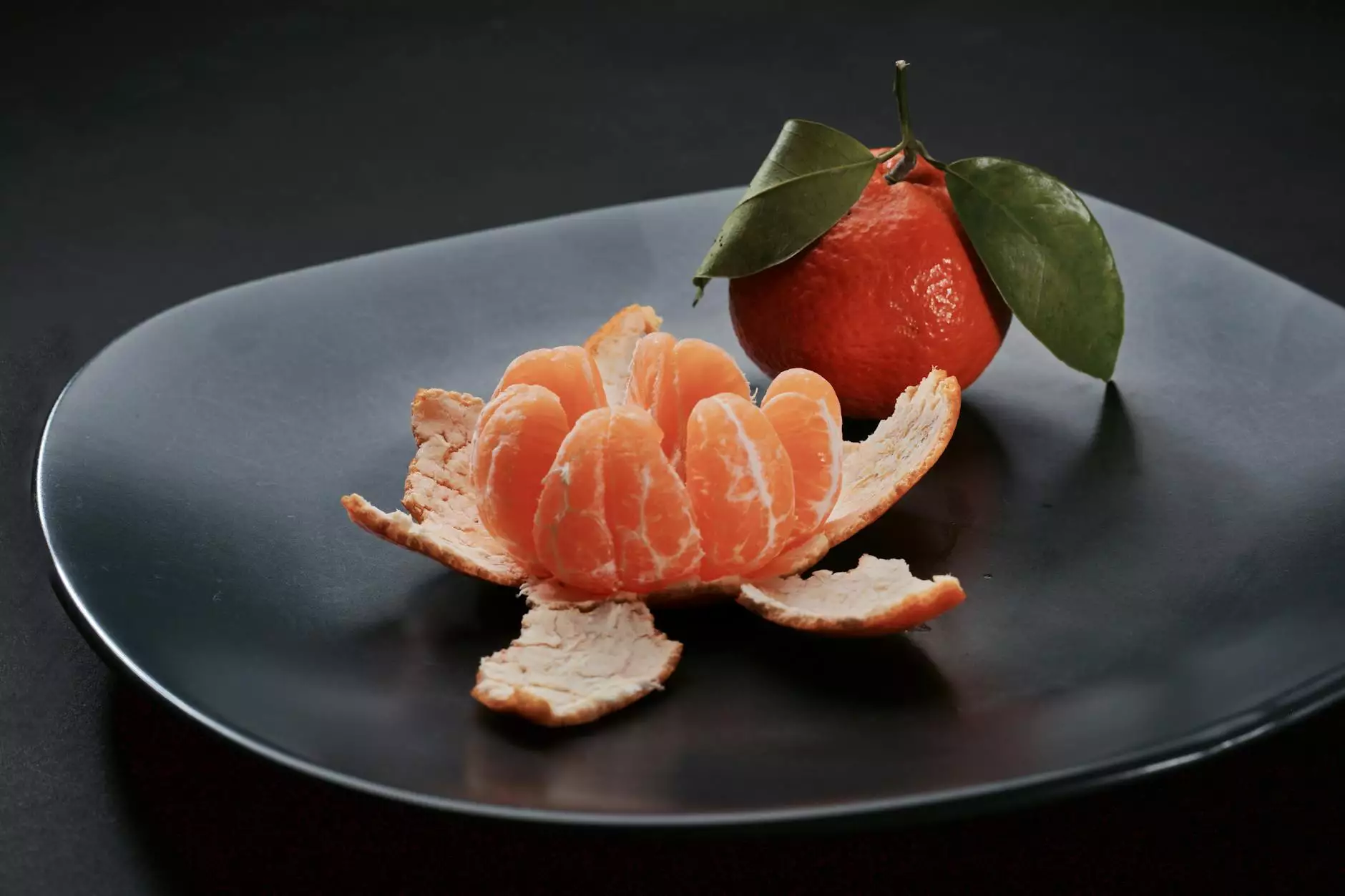
The rise of artificial intelligence (AI) and machine learning (ML) technologies has revolutionized various industries, making quality data more crucial than ever. Among the various methods of data annotation, semantic segmentation video stands out as a transformative approach. This article delves deep into the intricacies of semantic segmentation video, how it aligns with businesses like Keylabs.ai, and why it is the go-to solution for effective data annotation.
What is Semantic Segmentation?
Semantic segmentation is the process of classifying each pixel in an image into a category. Unlike traditional image classification, which categorizes an entire image, semantic segmentation elucidates the specific regions within the image that belong to particular classes. This technique is pivotal in applications like autonomous driving, medical imaging, and geographical analysis.
Why is Semantic Segmentation Important?
In today’s data-driven world, the demand for concepts like semantic segmentation rises sharply. The following points illustrate its importance:
- Precision: Every pixel is assessed, allowing for high precision in understanding scenes.
- Enhanced Data Quality: Offers better insights into the structure of objects within images, leading to higher-quality data for training algorithms.
- Application Versatility: Useful across multiple domains, including autonomous vehicles, robotics, and health diagnostics.
- Improved Decision Making: Provides in-depth analysis, empowering stronger decision-making processes.
The Impact of Video in Semantic Segmentation
When we integrate videos into the semantic segmentation process, we achieve a profound enhancement in how data is interpreted and utilized. Videos introduce the element of time, adding another dimension to data analysis. Here’s how semantic segmentation video is reshaping data annotation:
Temporal Contextualization
By analyzing consecutive frames in a video, machines can identify motion patterns and transitions that are invisible in static images. This ability leads to:
- Better Object Tracking: Objects can be tracked across frames, allowing for a more comprehensive understanding of their behavior.
- Contextual Awareness: Understanding the context in which an object appears enhances semantic meaning.
- Dynamic Scene Analysis: Enables the analysis of evolving scenarios, crucial for applications like surveillance.
How Semantic Segmentation Video Advances Data Annotation
Incorporating semantic segmentation into video analysis offers businesses unparalleled enhancements to their data annotation processes. Here’s how:
1. Accelerated Annotation Processes
Businesses utilizing Keylabs.ai’s data annotation tools can leverage automated segmentation techniques that expedite the annotation of video data. Automation reduces the time required for manual annotations, leading to:
- Increased Efficiency: Annotators can focus on verification rather than basic annotation tasks.
- Cost Savings: Streamlined processes lead to reduced labor costs.
2. Enhanced Accuracy
Machine learning algorithms, when trained on accurately labeled video data, can achieve remarkable accuracy levels. The advantages include:
- Reliable Outputs: Accurate pixel-level labeling results in better performance in downstream tasks.
- Fewer Errors: Reduces human error common in traditional annotation methods.
3. Richer Datasets
Video data inherently carries more information than still images. By integrating semantic segmentation, businesses can create:
- Detailed Training Sets: Rich video datasets improve machine learning model performance.
- Diverse Scenarios: Videos capture a wide range of contexts, enhancing model adaptability.
Challenges of Implementing Semantic Segmentation Video
Despite its advantages, implementing semantic segmentation in video analysis is not without challenges. Here are some common obstacles faced by businesses:
1. Resource Intensive
Semantic segmentation video requires substantial computational power and storage capacity due to the volume of data processed. Therefore:
- Investments in infrastructure may be necessary for companies looking to effectively implement these solutions.
- Cloud-based solutions can alleviate some of the burdens, allowing businesses to scale operations as needed.
2. Complexity of Models
The algorithms needed to perform semantic segmentation on video frames can be complex, often requiring:
- Advanced knowledge in machine learning and deep learning.
- Extensive training data to achieve competent model performance.
Keylabs.ai: Leading the Way in Data Annotation
Keylabs.ai is at the forefront of this technological evolution, providing businesses with a data annotation platform that harnesses the power of semantic segmentation video. Here’s how:
Comprehensive Tools and Solutions
The platform offers:
- User-Friendly Interfaces: Intuitive design enables easy navigation and swift onboarding.
- Scalability: Businesses can scale their annotation efforts according to their project needs, ensuring flexibility.
- Real-Time Collaboration: Teams can work synchronously, enhancing productivity and consistency in results.
Expert Support and Resources
Keylabs.ai provides:
- Access to AI Experts: Businesses can tap into a reservoir of knowledge to optimize their annotation processes.
- Regular Updates and Improvements: The platform continuously evolves to incorporate the latest advances in data annotation technology.
Conclusion: Embracing the Future of Data Annotation
As businesses increasingly turn to AI and ML, the demand for precise and efficient data annotation grows. Semantic segmentation video offers a robust solution, enhancing accuracy, accelerating processes, and providing richer datasets. Investing in innovative platforms like Keylabs.ai ensures businesses keep pace with technological advancements, leveraging data annotation tools that drive success. As we look to the future, embracing these methodologies will undoubtedly pave the way for groundbreaking developments in AI and beyond.